Data is the foundation of modern decision-making, but not all data is created equal. Poor-quality data can lead to wrong conclusions and costly mistakes. That’s why data quality management is critical—it ensures the data you rely on is accurate, complete, and trustworthy.
Whether you're working with customer information, financial reports, or operational metrics, understanding how to manage data quality effectively is key. This article breaks down what data quality management involves, its core elements, and why it matters.
Key Elements and Benefits of the Data Quality Management Process
Data quality management (DQM) is the foundation of any successful data-driven strategy. It ensures that the information fueling your decisions is reliable, consistent, and actionable. To achieve this, a robust DQM process includes several key elements: data profiling, cleansing, integration, and monitoring.
Each of these components plays a foundational role in maintaining high-quality data, helping organizations minimize errors and optimize their operations.
Data profiling involves analyzing datasets to find patterns, anomalies, and inconsistencies. By auditing data against metadata and quality benchmarks, you can identify potential issues before they escalate.
Data cleansing focuses on correcting errors, such as removing duplicates, filling in missing values, or standardizing formats. This prevents flawed data from compromising decision-making processes.
Data integration ensures data from disparate sources is combined into a unified system, resolving inconsistencies and redundancies along the way.
Data monitoring involves ongoing tracking of data quality metrics, enabling real-time identification of problems that require immediate action.
Clear data quality criteria, including accuracy, consistency, completeness, and timeliness, are essential for successful data management. These benchmarks ensure data reflects real-world facts, aligns across systems, contains all necessary fields, and is available when needed, forming the foundation for reliable data quality management processes.
Effective DQM provides significant benefits, from better decision-making and operational efficiency to regulatory compliance and customer satisfaction.
High-quality data enables accurate analyses, personalized customer experiences, and long-term trust in data-driven initiatives, positioning organizations for sustained success and reducing costly errors.
Best Practices for Effective Data Quality Management
To ensure high-quality data, organizations must adopt best practices that maintain accuracy, consistency, and usability, enabling better decision-making and operational efficiency. A strong data governance structure is essential, with clearly assigned roles like Data Stewards to oversee data as an asset and Program Managers to manage daily tasks. Without clear ownership, data quality efforts can become fragmented and ineffective.
Key practices include:
Establishing standardized metrics: Define criteria for accuracy, completeness, and timeliness to ensure consistency across systems.
Monitoring and auditing data: Regularly review datasets to identify and address issues such as inconsistencies, duplicates, or missing fields, preventing flawed data from impacting operations.
Fostering a quality-aware culture: Provide employee training on data entry and error recognition, empowering staff to maintain data integrity.
Technology is crucial for streamlining these efforts. Tools for data profiling and cleaning can automate tasks, such as identifying anomalies or resolving duplicate records, reducing manual errors, and enhancing efficiency.
These measures support regulatory compliance, lower risks, and improve long-term outcomes. By integrating governance, technology, and a quality-driven culture, organizations can establish a robust framework for data quality, driving better decisions and sustained growth.
Read our article "Top Data Quality Management Best Practices You Need to Know" to discover key strategies for maintaining high-quality data.
Top Data Quality Management Tools
Efficient data quality management requires advanced tools that ensure accuracy, consistency, and reliability, especially for organizations handling large data volumes. These tools streamline critical processes such as data profiling, cleansing, and monitoring.
Some tools like Talend, Informatica, and IBM InfoSphere offer diverse functionalities, from automation to enterprise-grade governance. However, successful implementation demands tools tailored to your needs, including scalability, compatibility, and integration ease.
eSystems excels in delivering Master Data Management (MDM) solutions that integrate with leading tools, enabling businesses to optimize data governance and quality. With expertise in technologies like Mendix, OutSystems, and Workato, eSystems offers end-to-end services, including data integration, automation, and digital transformation.
By utilizing their MDM expertise, organizations can automate repetitive tasks, reduce errors, and ensure up-to-date, reliable data for informed decision-making, driving operational efficiency and strategic success.
Key Components of a Data Quality Management Framework
A Data Quality Management (DQM) Framework provides a structured approach to ensure data accuracy, consistency, and reliability, forming the foundation of an effective data strategy.
Core components of a DQM Framework include:
Data Governance: Clearly defined roles, responsibilities, and accountability ensure everyone understands their part in maintaining data quality. For example, data stewards oversee dataset integrity, while analysts work with clean, valid data. Governance also establishes policies, enforces standards, and resolves conflicts.
Data Quality Dimensions:
Accuracy: Does the data reflect real-world facts?
Completeness: Are all necessary fields populated?
Consistency: Is the data uniform across systems?
Timeliness: Is the data current and accessible when needed?
Operational Processes:
Data profiling: Audit datasets for patterns and inconsistencies.
Data cleansing: Correct errors like duplicates or missing values.
Data validation: Verify adherence to rules before use.
Data monitoring: Continuously track and resolve issues.
Automated Data Quality Management
Automation is transforming data quality management by reducing manual effort, minimizing human errors, and enhancing efficiency and reliability.
Leveraging machine learning (ML) and artificial intelligence (AI), automated tools analyze datasets, detect patterns, and identify anomalies that may go unnoticed in manual reviews. For instance, AI can flag inconsistencies or irregularities in real-time, enabling proactive issue resolution.
Real-time data monitoring and correction are key advantages of automation, especially in dynamic environments or when handling high data volumes. Automated systems continuously scan data streams, instantly identifying and resolving errors to maintain quality in time-sensitive operations.
Additionally, automation scales seamlessly with increasing data complexity and size, ensuring consistent data quality as organizations grow.
To fully capitalize on automation, tools must align with organizational data quality standards and strategic goals. Configuring automated systems based on predefined rules and integrating them into a robust data governance framework ensures compliance and supports long-term objectives.
By modernizing processes through automation, organizations can uphold data quality standards efficiently, even as data demands expand.
Enterprise Data Quality Management
Managing data quality at an enterprise level involves addressing challenges arising from massive, diverse datasets generated across internal systems, customer interactions, and third-party sources.
This complexity can lead to inconsistencies and errors, requiring strategic approaches to maintain high-quality data organization-wide.
Cross-departmental collaboration is crucial to enterprise data quality management. Since data flows between departments like marketing, sales, and operations, coordinated efforts ensure consistency in definitions and standards, reducing discrepancies and fostering uniformity.
A centralized governance structure further supports this by setting policies, monitoring compliance, and enforcing accountability across all departments to meet regulatory and operational requirements.
Scalable tools and technologies are essential for handling the scope of enterprise data. Platforms with capabilities like real-time monitoring, automated cleansing, and anomaly detection streamline processes and prevent data quality issues from affecting critical operations.
Aligning data quality strategies with organizational goals, such as enhancing customer experience through accurate and complete customer data, ensures data quality becomes a driving force behind business success.
The Importance of Data Quality Management in Healthcare
Data quality management is crucial in healthcare, directly impacting patient safety and operational efficiency. High-quality data ensures accurate patient records, supporting precise diagnoses and effective treatments. In contrast, incomplete or inconsistent data can lead to serious consequences, such as misdiagnoses, medication errors, and inappropriate treatment decisions, jeopardizing patient safety.
Maintaining high data quality also helps healthcare organizations meet strict regulatory requirements like HIPAA and GDPR, ensuring data privacy and security. Compliance with these standards reduces the risk of penalties and protects sensitive patient information, fulfilling both legal and ethical obligations.
Reliable data quality further drives innovation in healthcare technologies, such as AI-driven diagnostics and personalized medicine, which depend on accurate and comprehensive data to function effectively. Operational efficiency also benefits from high-quality data by reducing redundancies, streamlining processes, and improving resource allocation, enabling providers to deliver better patient care and enhance overall organizational performance.
Read our article "Why Data Quality Management in Healthcare Matters" to learn more about the importance of data quality management in healthcare.
Best Practices for Ensuring Data Quality for Asset Management
Maintaining accurate and consistent data is essential for effective asset management, enabling better resource allocation, maintenance planning, and forecasting. Without reliable data, decision-making becomes error-prone, leading to inefficiencies and missed opportunities.
Key Best Practices:
Standardized Data Collection and Entry:
Implement predefined templates or fields for asset details (e.g., serial numbers, purchase dates, and maintenance schedules).
Ensure uniformity in recording asset-related information to minimize errors caused by inconsistent formats or manual mistakes.
Regular Data Audits and Validations:
Conduct periodic audits to identify and correct errors such as duplicates or incomplete records.
Maintain an up-to-date dataset to ensure accurate decision-making and prevent issues from escalating.
Integration into Asset Lifecycle Management:
Verify data accuracy at every stage, from procurement to disposal.
Prevent data inaccuracies during procurement to avoid errors affecting later stages, like maintenance or decommissioning.
Utilization of Technology:
Use asset management software to automate data validation, anomaly detection, and reporting.
Leverage real-time monitoring features to identify and address data issues promptly, reducing manual workload and improving accuracy.
Read our article "How to Ensure Proper Data Quality for Asset Management" to learn more about maintaining accurate and reliable data for asset management.
Strategies to Enhance Financial Data Quality Management in Your Organization
Improving financial data quality management is vital for accurate reporting, informed decision-making, and regulatory compliance. Organizations can achieve this by adopting structured strategies that address challenges and align with business goals.
Key Strategies:
Implement Robust Validation and Reconciliation:
Use data validation and reconciliation processes to detect errors and ensure integrity.
Cross-check transactions between general ledgers and sub-ledgers or reconcile bank statements to prevent discrepancies.
Establish Data Ownership and Accountability:
Assign roles like data stewards or financial controllers to oversee data quality.
Promote accountability by enforcing governance policies and ensuring compliance across departments.
Utilize Financial Data Management Tools:
Use ERP systems, data quality software, or automation platforms for tasks like standardization, anomaly detection, and duplicate removal.
Automate repetitive processes to reduce errors and free up resources for strategic tasks.
Foster a Culture of Data Quality Awareness:
Educate finance teams on the importance of data quality and its impact.
Provide regular training, communicate best practices, and integrate data quality metrics into performance evaluations to encourage proactive efforts.
Take the Next Step in Improving Your Data Quality Management
Ensuring robust data quality management is no longer optional—it’s a necessity for maintaining competitive advantage and driving informed decision-making. At eSystems, we specialize in enabling businesses like yours to implement effective, automated solutions tailored to your unique challenges.
Whether you're looking to integrate low-code technologies for seamless automation or need guidance in building a comprehensive data management framework, we’re here to help. Visit our Contact Us page to start the conversation. Let’s work together to transform your data into a strategic asset.
FAQ
What Are the 5 Rules of Data Quality?
The five rules of data quality are accuracy, completeness, consistency, timeliness, and validity, ensuring reliable, comprehensive, uniform, current, and standards-aligned data.
What Are the Four Pillars of Data Quality Management?
The four pillars of data quality management are accuracy, completeness, consistency, and timeliness, forming the core principles for maintaining reliable and actionable data.
What Are the 6 C's of Data Quality?
The 6 C's of data quality—completeness, consistency, conformity, accuracy, currency, and credibility—ensure data is comprehensive, uniform, standards-compliant, accurate, up-to-date, and trustworthy.
What Is the DQM Process?
The DQM process involves monitoring, assessing, and improving data to meet standards for accuracy, completeness, consistency, timeliness, and reliability.
Why Is Data Quality Important?
Data quality is important because it enables accurate decisions, operational efficiency, customer satisfaction, regulatory compliance, and trust in data-driven processes.
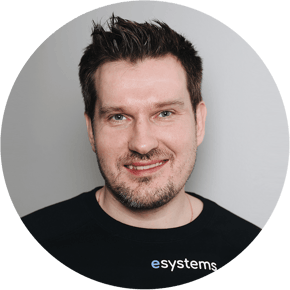
COMMENTS