Data is the foundation of decision-making, but even the best tools and analytics are only as good as the quality of the data you feed them. Poor data quality leads to errors, inefficiencies, and missed opportunities.
Managing data effectively is no longer optional—it’s a necessity for staying competitive in today’s fast-paced business environment. This article outlines key best practices for improving and maintaining high-quality data.
Gain a better understanding of data quality management by reading our article "Understanding Data Quality Management: A Complete Guide."
What Is Data Quality Management?
Data quality management ensures that information is accurate, consistent, and reliable for its intended use. It involves strategies, processes, and tools to address errors, inconsistencies, duplicates, and outdated data throughout its lifecycle—from collection to storage and analysis. High-quality data supports informed decision-making, enhances customer experiences, and ensures compliance, while poor data can lead to costly business losses.
Managing data quality is challenging due to increasing data volumes from diverse sources like cloud platforms and IoT devices, which introduce inconsistencies and errors. Additionally, AI and machine learning require clean, reliable data to generate meaningful insights. Organizations must implement validation, cleansing, and continuous monitoring to maintain data integrity.
Ultimately, strong data quality management builds trust in data, enabling businesses to leverage it as a strategic asset. Without it, even the most advanced analytics tools lose effectiveness, leading to flawed insights and operational inefficiencies.
Best Practices for Data Quality Management
1. Define Clear Data Quality Goals
To effectively manage and improve data quality, start by setting clear, measurable goals aligned with your organization’s broader objectives. These goals provide direction and ensure that data quality initiatives contribute to strategic business outcomes. For instance, if the aim is to improve customer satisfaction, focus on enhancing the accuracy and completeness of customer records.
Key data quality dimensions to address include:
Accuracy: Ensuring data correctly represents real-world facts.
Consistency: Maintaining uniformity across datasets to prevent discrepancies.
Completeness: Filling in missing values to create a fully usable dataset.
Collaboration across departments—such as IT, marketing, and operations—is essential to align priorities and ensure broad support. Stakeholder engagement fosters accountability and helps address real-world data challenges.
2. Develop a Data Governance Framework
A data governance framework ensures order, accountability, and consistency in data management. Without it, data processes can become fragmented, leading to inefficiencies and costly errors.
The first step in creating a governance framework is defining clear roles and responsibilities. Data stewards, analysts, and executives must understand their duties—such as overseeing data quality, enforcing policies, and aligning practices with business goals. This ensures accountability and prevents oversight.
The framework should also establish policies and standards for data management, covering areas like access controls, data retention, and formatting. These guidelines ensure uniformity and reduce ambiguity.
Since business needs evolve, regularly reviewing and updating the governance framework is essential. This keeps it relevant and aligned with changing regulations, technologies, and organizational priorities.
3. Standardize Data Collection and Entry
To maintain accurate, reliable, and useful data, standardizing data collection and entry methods is essential. Without consistent practices, errors and inconsistencies can undermine data integrity and impact decision-making. Implementing best practices for standardization minimizes risks and ensures seamless data integration and analysis.
Key Steps for Standardizing Data Entry:
Establish Clear Procedures: Define standardized processes for data collection and entry and ensure consistency in capturing essential details like full names, addresses, and contact information.
Provide Training and Guidelines: Educate personnel on data entry standards and best practices and use training sessions and reference materials to ensure adherence and accountability.
Use Predefined Data Formats and Templates: Set formats for dates (e.g., "YYYY-MM-DD") and phone numbers (with country codes).
Implement Validation Rules and Automated Checks: Detect incomplete fields, invalid email addresses, or incorrect data types and automate error detection to reduce manual mistakes and improve efficiency.
4. Utilize Data Quality Tools and Automation
To maintain high-quality data, leveraging data quality tools and automation is essential. These solutions streamline processes, minimize errors, and ensure consistency across datasets, reducing manual effort while maintaining data integrity.
Benefits of data quality tools and automation:
Automates Repetitive Tasks: Handles data cleansing, validation, and deduplication and reduces human error and frees resources for strategic initiatives.
Provides Real-Time Monitoring: Detects and resolves data quality issues as they arise and prevents minor problems from escalating into costly challenges.
Scales Data Quality Efforts: Manages large datasets efficiently without overwhelming teams and ensures consistency across multiple systems.
Enhances Resource Allocation: Allows teams to focus on governance, analytics, and innovation.
5. Perform Regular Data Audits and Assessments
Regular data audits and assessments are essential for maintaining high-quality, reliable data. These evaluations help detect and address issues before they escalate, preventing inaccuracies, inconsistencies, and errors that could impact decision-making and efficiency.
Benefits of data audits:
Identify Patterns and Issues: Detect formatting errors, missing fields, and duplicate records and address root causes to prevent recurring data problems.
Ensure Compliance: Verify alignment with data governance policies and industry standards and maintain trust and accountability within the organization.
Guide Targeted Improvements: Use audit findings to refine processes, update procedures, and enhance validation rules and implement automation to reduce manual errors and improve accuracy.
Track and Document Progress: Maintain a record of issues, corrective actions, and improvements over time and foster a culture of continuous data quality enhancement.
Regular audits help mitigate risks, improve operational efficiency, and support better business decisions.
6. Establish Data Quality Metrics
Establishing data quality metrics is essential for measuring the effectiveness of data management efforts. These metrics provide a standardized way to track progress, identify weaknesses, and ensure accountability. Without clear metrics, it’s difficult to assess whether data quality initiatives align with business goals or deliver meaningful improvements.
To define effective metrics, organizations should focus on key data quality dimensions, often summarized as accuracy, consistency, completeness, integrity, and timeliness. Accuracy ensures data correctly represents real-world facts, while consistency verifies uniformity across systems.
Completeness confirms that no critical data points are missing, integrity maintains relationships between data elements, and timeliness measures how quickly data becomes available when needed.
Different industries prioritize different metrics. Customer-facing sectors emphasize accuracy and completeness, while time-sensitive industries like healthcare focus on timeliness. Operational efficiency efforts may track duplicate records and error rates.
Visual dashboards and reports help monitor these metrics, providing real-time insights that guide continuous improvement and better decision-making.
7. Implement Master Data Management
Master Data Management (MDM) enhances data consistency and reliability by centralizing key information such as customer records, product details, and supplier data.
By establishing a single, authoritative source of truth, MDM eliminates redundancies, resolves discrepancies, and ensures all departments work with accurate, up-to-date information.
A major benefit of MDM is reducing data conflicts. Without a centralized system, different teams may use duplicate or inconsistent data. MDM consolidates core business information, improving efficiency and trust in data.
MDM also strengthens decision-making. Accurate data enables better customer segmentation, optimized inventory management, and precise financial forecasting. To ensure success, clear ownership and governance structures—such as data stewards—must be established. This accountability maintains long-term data quality.
Additionally, MDM improves system integration by standardizing data formats, making it easier to merge datasets or synchronize information across departments. This seamless data-sharing enhances collaboration and operational efficiency across the organization.
eSystems provides specialized MDM services, offering expertise in implementing robust master data management solutions that enhance data quality, integration, and governance for enterprises.
8. Ensure Data Security and Compliance
Protecting your organization’s data is essential for maintaining trust, preventing breaches, and safeguarding your reputation. Sensitive data, including customer information, financial records, and proprietary business data, must be secured to prevent unauthorized access and misuse. Failure to do so can result in financial losses and long-term damage to stakeholder confidence.
Compliance with regulations like GDPR, HIPAA, and CCPA is crucial. Non-compliance can lead to penalties and legal issues, while adherence reinforces accountability and trust. Implementing robust access controls and encryption enhances security by restricting access and protecting data from cyber threats.
Regular security audits and risk assessments help identify vulnerabilities and ensure security measures remain effective. Employee training on security protocols further reduces risks by minimizing human errors.
9. Involve Business Users in Data Quality Initiatives
Engaging business users in data quality initiatives ensures alignment with operational realities and enhances practical outcomes. While data teams handle technical aspects, business users provide valuable insights into daily data usage, bridging the gap between technology and real-world applications. Their involvement makes data quality initiatives more relevant and effective.
With domain expertise, business users can identify critical issues, such as discrepancies in customer records affecting sales or missing product data limiting marketing efforts. Their input helps organizations prioritize impactful areas rather than wasting resources on less significant data issues.
Involving business users also fosters ownership and accountability. When they see their feedback reflected in improved systems, they become more invested in maintaining data integrity. This shared responsibility lightens the load on data teams and ensures consistency across departments.
Collaboration between business users and data teams leads to actionable solutions that are both technically sound and operationally viable. Regular feedback loops help organizations continuously refine data quality strategies to meet evolving business needs.
10. Create a Data Quality Culture
Building a strong data quality culture ensures that maintaining high-quality data is a shared responsibility across the organization rather than a siloed task. When data quality becomes a core value, it enhances decision-making, operational efficiency, and trust in data.
Leadership’s Role: Executives must prioritize data quality by allocating resources, setting policies, and embedding it into strategic goals. Visible commitment from leadership signals its importance to all employees.
Continuous Education and Training: Employees need ongoing training on data quality best practices. Workshops, tool training, and accessible resources help reinforce its significance and prevent costly errors.
Shared Responsibility Across Teams: Since every department interacts with data, collaboration is essential. Cross-functional teams, such as marketing and IT, should define data standards together, while sales ensures accurate data input.
Recognition and Incentives: Acknowledging teams that maintain high data standards fosters engagement. Public recognition, awards, or incentives reinforce the importance of data quality.
11. Enable Continuous Improvement
Continuous improvement is essential for maintaining effective data quality management. As organizational goals, technology, and market conditions evolve, data quality processes must be regularly refined to stay relevant. Without ongoing updates, even high-quality data can become outdated or inaccurate.
Regular reviews help identify emerging challenges, such as compliance changes or shifts in data usage. Evaluating workflows and tools ensures they align with business needs. Monitoring key metrics—like accuracy, consistency, and timeliness—reveals trends and highlights areas needing improvement. User feedback also provides valuable insights into practical data challenges that automated systems may overlook.
Learning from past data quality projects enhances future efforts. Successful techniques should be expanded, while ineffective strategies must be revised. Keeping up with advancements in AI, machine learning, and low-code platforms helps automate quality checks and streamline processes.
Take the Next Step Toward Better Data Quality Management
Implementing effective data quality management practices is important for driving organizational success and ensuring reliable decision-making. At eSystems, we specialize in solutions like Master Data Management and automation tools to help businesses like yours achieve data quality excellence.
If you're ready to elevate your data management strategy or need guidance tailored specifically to your organization, contact our team today. Let us help you transform your data into a strategic asset.
FAQ
What Are the 7 C's of Data Quality?
The 7 C's of data quality are completeness, consistency, correctness, currency, clarity, credibility, and connectivity.
What Are Data Quality Best Practices?
Data quality best practices include establishing clear data governance policies, implementing data validation processes, ensuring consistent data formatting, and promoting a culture of data stewardship.
What Are the 5 Principles of Data Quality?
The five principles of data quality are accuracy, completeness, consistency, timeliness, and validity.
What Are the Four Pillars of Data Quality Management?
The Four Pillars of Data Quality Management are accuracy, completeness, consistency, and timeliness.
What Is Data Quality Management (DQM)?
Data Quality Management (DQM) is the process of ensuring data is accurate, consistent, complete, and reliable throughout its lifecycle.
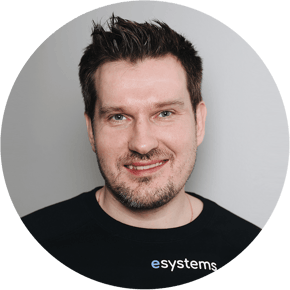
COMMENTS