Asset management decisions are only as good as the data behind them. Poor data quality can lead to costly errors, missed opportunities, and inefficiencies. So, how do you ensure your data is accurate, reliable, and actionable? This article breaks down what data quality really means for asset management and how to implement it effectively.
For more insights on data quality management, read our article "Understanding Data Quality Management: A Complete Guide."
What Is Data Quality in Asset Management?
Data quality in asset management is ensuring that the information used to track, maintain, and optimize assets—both physical and digital—is accurate, consistent, and reliable. High-quality data forms the foundation for effective asset management, enabling organizations to make informed decisions, mitigate risks, and allocate resources efficiently.
High-quality data in asset management exhibits several key characteristics:
Accuracy: The data have to reflect the true state of the assets it describes, free from errors or misleading information.
Completeness: All relevant fields and values have to be present, with no critical gaps that could restrict decision-making.
Timeliness: Up-to-date data ensures that decisions are based on current information instead of outdated records.
Consistency: Uniform formatting, standards, and rules across systems prevent discrepancies and confusion.
Relevance: The data have to serve its intended purpose, directly supporting asset-related decisions and strategies.
When these characteristics are met, data quality becomes a powerful enabler of informed decision-making. For example, accurate and timely data allows you to analyze asset performance effectively, plan maintenance schedules, and make investment decisions with confidence.
For organizations seeking to elevate their data governance practices, solutions like those offered by eSystems can be transformative. Their MDM services provide a comprehensive approach to managing asset data, from ensuring compliance with established policies to integrating data from multiple departments into a single, reliable source of truth.
Steps to Ensure Proper Data Quality for Asset Management
1. Establish a Data Governance Framework
A well-defined data governance framework is essential for ensuring data quality in asset management by providing structure, accountability, and consistency.
Define Policies, Roles, and Responsibilities: Assign roles like data stewards to enforce quality standards and data owners to oversee data health. Develop policies that ensure accuracy, consistency, and completeness in data management.
Foster Collaboration Across Departments: Align teams such as IT, operations, and finance to prevent data silos and ensure a unified approach to data quality.
Establish and Enforce Standards: Create rules for data formatting and validation to ensure consistency and reliability across the organization.
Conduct Regular Reviews and Updates: Adapt the framework to evolving organizational needs, regulatory changes, and new technologies through periodic evaluations and updates.
2. Leverage Technology
Technology is vital for maintaining data quality in asset management by automating processes, minimizing errors, and enhancing efficiency. Manual data management is time-consuming and error-prone, while technological solutions streamline tasks and enforce accuracy. Dedicated tools, such as asset management software, apply validation rules, standardize formats, and flag errors like duplicates or missing fields, ensuring consistent and reliable data.
Real-time monitoring further enhances reliability, allowing organizations to identify and resolve issues as they arise, reducing the risks of outdated or inconsistent data. Advanced technologies like AI and machine learning improve these efforts by detecting patterns, predicting recurring issues, and enabling proactive mitigation, while also reducing manual workload.
Cloud-based solutions play a key role in breaking down data silos, offering centralized systems for seamless collaboration and access. By leveraging these technologies, organizations ensure consistent, accurate, and actionable data, driving better decision-making and operational efficiency.
3. Conduct Regular Data Audits
Conducting regular data audits is important for maintaining high-quality data in asset management.
It ensures the following:
Proactive Issue Identification: Audits uncover errors, inconsistencies, and gaps in data before they impact decision-making.
Quality Validation: Ensure data is accurate, complete, and up-to-date, supporting effective planning and forecasting.
Trend Analysis: Identify recurring issues (e.g., frequent errors during data entry) and address root causes.
Documentation: Maintain historical audit records to monitor progress, guide strategies, and ensure accountability.
4. Standardize Data Entry
Standardizing data entry ensures consistency and accuracy in asset management by reducing errors and aligning information across systems. Uniform processes prevent issues like mistyped values, incomplete fields, or format discrepancies caused by varied input practices.
Clear data entry guidelines and templates simplify the process, specifying required fields, acceptable formats, and validation rules to minimize mistakes. Regular staff training reinforces these standards, ensuring compliance and improving data reliability.
By adopting a standardized approach, organizations create a solid foundation for accurate and reliable asset management, enhancing decision-making and operational efficiency while reducing risks associated with inconsistent data practices.
5. Integrate Systems
System integration is essential for maintaining high data quality in asset management, as it eliminates silos and promotes seamless data sharing.
Eliminate Data Silos: Independent systems create fragmented and inconsistent information. Integrating systems ensures consistency by enabling unified data access across platforms.
Centralize Data: Consolidate information from multiple sources into a single system for comprehensive asset tracking, management, and analysis. Centralization prevents duplication, errors, and inefficiencies.
Real-Time Synchronization: Integrated systems update and reflect changes instantly across all connected platforms, ensuring everyone uses the most current data.
Foster Collaboration: Reliable, consistent data supports better teamwork and informed decision-making by reducing miscommunication and aligning departments.
Support Scalability: As asset management needs grow, adaptable and scalable systems maintain data quality, even with expanding operations or new technologies.
6. Monitor and Improve Continuously
Maintaining data quality requires consistent oversight and a commitment to ongoing improvement as systems and organizational needs evolve.
Establish KPIs: Define metrics for accuracy, completeness, and consistency to assess data health. Regular reviews of these indicators help identify patterns and address issues early.
Create Feedback Loops: Collect user and system feedback to identify gaps or inefficiencies, informing targeted improvements such as refining processes or adding validation tools.
Foster a Culture of Improvement: Encourage teams to view data quality as an ongoing responsibility through regular reviews, training, and the adoption of best practices.
Adapt to Change: Use insights from monitoring efforts to align processes with evolving technologies, regulations, and organizational goals. Regular analysis ensures proactive adjustments.
Best Practices for Implementing Data Quality in Asset Management
1. Automate Where Possible
Automation is crucial for maintaining high-quality data in asset management by minimizing manual intervention and reducing human error. Automated processes ensure consistency and precision in data handling, improving reliability.
Efficiency is another key benefit—tasks like data validation and cleansing can be performed faster, freeing up resources for strategic initiatives. Additionally, automated systems are scalable, and capable of processing large datasets without compromising accuracy, making them ideal for growing organizations with increasing data complexity.
Real-time monitoring is another advantage of automation, enabling instant alerts for issues like missing or inconsistent data. This allows for immediate corrective action, preventing small errors from escalating into significant problems. Automation also ensures uniform application of data quality standards, promoting consistency across operations.
2. Focus on Real-Time Data
Real-time data is essential in asset management, offering up-to-date insights that enhance decision-making and responsiveness. Access to current asset data allows organizations to quickly adapt to operational changes, minimizing downtime and optimizing utilization. Real-time data is particularly valuable for predictive maintenance, as it enables early detection of performance anomalies and potential failures, reducing costs and avoiding disruptions.
By continuously monitoring assets, organizations can promptly address underperformance or reallocate workloads to maintain efficiency. Real-time data also supports proactive risk management, identifying and mitigating issues such as equipment malfunctions or compliance risks before they escalate.
This immediacy improves operational efficiency, asset longevity, and overall risk management. Organizations that leverage real-time data gain a competitive edge by making informed decisions, optimizing maintenance schedules, and ensuring smooth operations.
3. Engage Stakeholders
Engaging stakeholders is vital for improving data quality in asset management. Involving relevant teams ensures data quality efforts align with organizational goals, creating a shared understanding of how data drives business outcomes. Cross-functional collaboration, including input from operations, IT, and finance, ensures that diverse data needs are met and challenges specific to asset management are addressed.
Stakeholder engagement fosters accountability and shared responsibility. When employees understand their role in maintaining data quality, they are more likely to follow best practices, reducing errors and improving consistency. Collaboration also helps identify practical and effective solutions, as stakeholders who interact with data daily can highlight inefficiencies and gaps.
4. Adopt a Scalable Approach
To maintain data quality as your organization grows or becomes more complex, adopting a scalable approach Is important. Without scalability, processes that work well today might fail under the strain of increasing data volume or diversity, leading to inefficiencies and inconsistent data quality.
Scalable data quality processes are designed to grow with your organization. They account for future expansions, whether that’s more assets, new data sources, or additional teams requiring access. For example:
Flexible systems enable you to adjust workflows as asset management requirements evolve. This might include updating data validation rules or integrating new tools without disrupting existing operations.
Standardized frameworks ensure consistency even as data complexity increases, making it easier to maintain quality across all organizational levels.
Ready to Elevate Your Data Quality Strategy?
Maintaining high data quality is no longer optional—it's important for effective asset management and long-term business success. At eSystems, we specialize in helping organizations like yours implement robust solutions for data quality through innovative digital change services.
Whether you're looking to establish a data governance framework, integrate systems seamlessly, or utilize automation for consistency, our expertise in low-code technologies and master data management can guide you every step of the way.
If you're ready to take the next step toward better data quality, contact our experts today to discuss how we can help. Or look into how our master data management solutions can enable your organization to achieve clarity and accuracy. The future of asset management starts with data you can trust—let’s build it together.
FAQ
What Are the 5 Elements of Data Quality?
The five elements of data quality are accuracy, completeness, consistency, timeliness, and reliability.
Why Is Data Important in Asset Management?
Data is important in asset management as it drives informed decision-making, optimizes performance, reduces costs, and ensures regulatory compliance.
What Are the 7 Factors of Data Quality?
The 7 factors of data quality are accuracy, completeness, consistency, timeliness, validity, uniqueness, and integrity.
What Are the 5 Data Quality Dimensions?
The five key data quality dimensions are accuracy, completeness, consistency, timeliness, and validity.
What Are Some Common Data Quality Issues in Asset Management?
Common data quality issues in asset management include incomplete or missing data, inconsistent formatting, outdated or inaccurate information, duplicate records, and lack of standardization across systems.
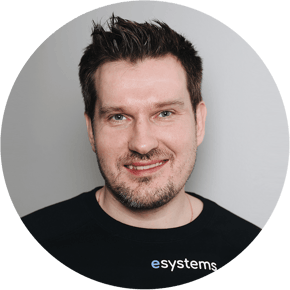
COMMENTS