Master Data Management (MDM) used to be a manual and time-consuming process. Today, AI is making it faster, smarter, and more efficient. From eliminating duplicates to improving data accuracy, AI is reshaping how companies handle their most critical information. Understanding how AI fits into MDM can help organizations improve workflows and make better business decisions.
This article explains what AI in Master Data Management is, why it matters, and the key benefits it offers.
What Is AI in Master Data Management?
AI in Master Data Management (MDM) enhances how organizations manage essential business information, such as customer, product, and supplier data. By applying AI, companies can improve data accuracy, consistency, and integration across departments and systems. AI helps automate labor-intensive tasks in traditional MDM, like data cleaning and maintenance, reducing manual intervention and the risk of human error. This allows organizations to maintain reliable, high-quality data with less effort, freeing up resources for more strategic activities.
A significant aspect of AI in MDM is machine learning, which identifies patterns, anomalies, and relationships within datasets. These algorithms can recognize customer behavior trends, flag inconsistencies, and suggest resolutions for data discrepancies, leading to more informed decision-making. Additionally, AI-powered tools continuously monitor data quality and predict future needs based on usage patterns, enabling automatic updates. This ongoing data maintenance ensures that organizations have accurate, current, and dependable data without requiring constant manual oversight, making MDM more efficient and effective.
Purpose of AI in Master Data Management
One of the primary goals of AI in MDM is to automate repetitive data management tasks. This reduces the need for manual intervention, which typically is time-consuming and error-prone. AI can handle tasks such as:
Identifying and merging duplicate records
Updating data based on predefined rules
Validating data entries according to existing standards
As a result, organizations can allocate fewer resources to manual data handling, increasing overall efficiency.
Additionally, AI helps in better decision-making by analyzing data patterns and trends. It can find insights in your master data that would be difficult for a human to identify. These insights can then guide business strategies, such as:
Optimizing supply chains
Improving customer experiences
Improving operational efficiency
Another key objective is continual monitoring and updating of master data. AI tools can track changes in real-time, ensuring that your data remains accurate and up-to-date. This is important for organizations dealing with rapidly changing datasets, where outdated information can lead to costly errors.
Key Benefits of AI in Master Data Management
1. Automated Data Processing
AI is transforming how master data is processed by automating repetitive tasks that previously required human intervention. This automation allows businesses to handle large volumes of data more efficiently, reducing the time and effort spent on manual tasks.
One of the key ways AI improves automated data processing is through its ability to manage routine tasks with greater speed and accuracy. For example, AI can:
Validate data to ensure it meets predefined standards
Cleanse data by identifying and correcting errors
Classify data into appropriate categories
AI also processes large amounts of master data in real time, which increases operational efficiency.
2. Improved Data Quality and Accuracy
AI continuously monitors data to keep it accurate, relevant, and up-to-date without manual intervention. This real-time monitoring catches and addresses issues like outdated or incorrect data, reducing the risk of inaccuracies spreading across systems. AI also detects and corrects errors, inconsistencies, and duplicates, especially when data from various sources is integrated. This automated error correction improves the quality of master data, leading to more reliable decision-making.
In addition, AI learns from historical patterns to predict and prevent future issues. By analyzing past errors, it can identify potential risks and take preemptive actions to avoid recurring problems. AI-powered data validation further enforces consistency by ensuring entries meet specific rules and standards. Automating complex data-cleansing tasks minimizes human error, ensuring data remains clean and reliable while allowing teams to focus on strategic priorities.
3. Enhanced Data Integration
AI enhances data integration within organizations by automating the process of combining data from multiple sources, reducing the need for manual effort. It automatically maps and harmonizes data from different systems, improving consistency and enabling faster, more efficient data unification. This is especially useful for organizations that pull data from various departments or external sources, as AI enforces standardized formats, making the data easier to analyze and act on.
Additionally, AI’s adaptability allows it to quickly adjust to new or changing data sources, minimizing downtime and disruption. This flexibility is vital in industries where data sources frequently evolve. AI also simplifies the integration of both structured and unstructured data, providing a more complete view of organizational data and leading to richer insights that traditional methods struggle to achieve.
For companies looking to implement seamless data integration, eSystems offers tailored Master Data Management solutions to help harmonize and consolidate data from diverse sources, ensuring a consistent and reliable data foundation.
4. Better Data Governance and Compliance
AI is instrumental in streamlining data governance and compliance for organizations managing large volumes of master data. By automating governance processes, AI enforces rules and standards consistently, ensuring that data adheres to internal policies without requiring extensive manual effort. This automation helps maintain data consistency and reduces the workload associated with compliance.
In addition to internal governance, AI continuously monitors data for compliance with external regulations, reducing the risk of fines or legal issues. AI systems can adapt to evolving regulatory requirements in real time, ensuring that data remains aligned with the latest standards.
AI also enhances transparency and traceability, logging every data action for easy verification during audits. By automating routine compliance tasks, AI frees up teams to focus on more strategic governance initiatives, boosting overall efficiency.
5. Predictive Data Quality Monitoring
AI enhances master data quality by using predictive capabilities to identify potential issues before they arise. By analyzing historical data patterns, AI detects trends and anomalies that may lead to problems like inconsistency and duplication.
This proactive monitoring allows organizations to address errors in real time, preventing them from escalating and impacting operations. With AI’s continuous learning from past irregularities, the system becomes more effective over time, reducing the need for costly, reactive fixes and ensuring data remains accurate, consistent, and reliable.
Strategies to Integrate AI into Master Data Management
Successfully leveraging AI in Master Data Management (MDM) requires thoughtful planning and implementation. Here are 3–5 strategies to help organizations effectively integrate AI into their MDM processes:
Start with Data Assessment and Preparation
Before integrating AI, assess the current state of your data. Identify gaps, inconsistencies, and redundancies. Properly preparing your data ensures that AI algorithms have high-quality input for training and analysis. This foundational step enhances the effectiveness of AI-driven MDM solutions.Implement AI-Driven Data Cleansing Tools
Use AI-powered tools to automate data cleansing tasks, such as deduplication, error detection, and correction. These tools can process vast amounts of data more efficiently than traditional methods, ensuring that only clean, accurate data enters your systems.Leverage Machine Learning for Data Matching
Machine learning algorithms can identify patterns and relationships within data, improving data matching and entity resolution. For example, AI can help match customer profiles from different systems, ensuring consistency across departments.Adopt Predictive Analytics for Proactive Management
Integrating predictive analytics enables proactive monitoring and management of master data. AI can forecast potential data issues, such as inconsistencies or duplications, allowing organizations to address problems before they escalate.Use Low-Code Platforms for Custom AI Integration
Deploy low-code platforms like Mendix or OutSystems to integrate AI into your existing MDM systems. These platforms allow businesses to build custom solutions quickly and adapt AI functionalities to specific needs, minimizing disruption during implementation.
Challenges of AI in Master Data Management
While AI brings transformative capabilities to Master Data Management, it’s not without its challenges. Understanding these obstacles is critical for planning a successful implementation:
Data Quality Issues
Poor-quality data can undermine AI’s effectiveness, as AI models rely heavily on accurate and consistent input. If the initial data is flawed, the AI outputs may be unreliable, leading to incorrect decisions.Integration Complexity
Integrating AI into existing MDM systems can be complex, especially for organizations with legacy systems or decentralized data structures. Ensuring seamless integration across multiple platforms requires advanced technical expertise.Cost and Resource Requirements
Implementing AI solutions for MDM can involve significant initial investments in tools, infrastructure, and training. Smaller organizations may find these costs challenging to manage.Regulatory Compliance Concerns
AI-driven data management must comply with various regulations, such as GDPR or CCPA. Ensuring AI processes align with these requirements adds an extra layer of complexity to the implementation.Skill Gaps and Change Management
Successful adoption of AI in MDM requires a skilled workforce familiar with AI technologies and data science. Additionally, managing organizational resistance to AI-driven workflows can be challenging.
Addressing these challenges involves a combination of robust planning, investment in training, and leveraging expert support to ensure smooth AI adoption and maximize its potential benefits in Master Data Management.
Ready to Utilize AI for Your Master Data Management Strategy?
As AI continues to redefine how businesses manage their master data, understanding its impact is important for staying competitive. Whether it's improving data quality, automating processes, or ensuring compliance, AI offers tangible benefits that can transform your data management practices.
If you’re ready to look into how AI can improve your data management, contact our team at eSystems. With our expertise in low-code development and business process automation, we can help you streamline your operations and unlock the full potential of your data.
FAQ
How Is AI Used in Master Data Management?
AI in Master Data Management automates data cleaning, matching, and integration, enhancing data quality and consistency across systems.
How Can AI Be Used in Data Management?
AI automates data cleansing and classification in Master Data Management, improving quality and accuracy and reducing manual work.
Is Master Data Management Outdated?
Master Data Management is evolving, with AI enhancing accuracy, real-time insights, and intelligent recommendations for greater efficiency.
How to Use AI for Data Governance?
AI automates data quality checks, compliance, and anomaly detection, streamlining classification, lineage tracking, and policy enforcement.
What Are the Benefits of Using AI in Master Data Management?
AI improves data accuracy, automates cleansing, enhances decision-making, reduces errors, and enables predictive analytics in Master Data Management.
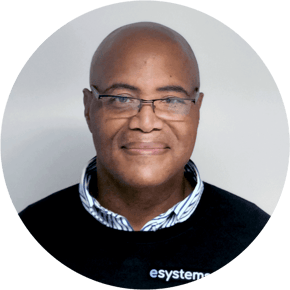
COMMENTS